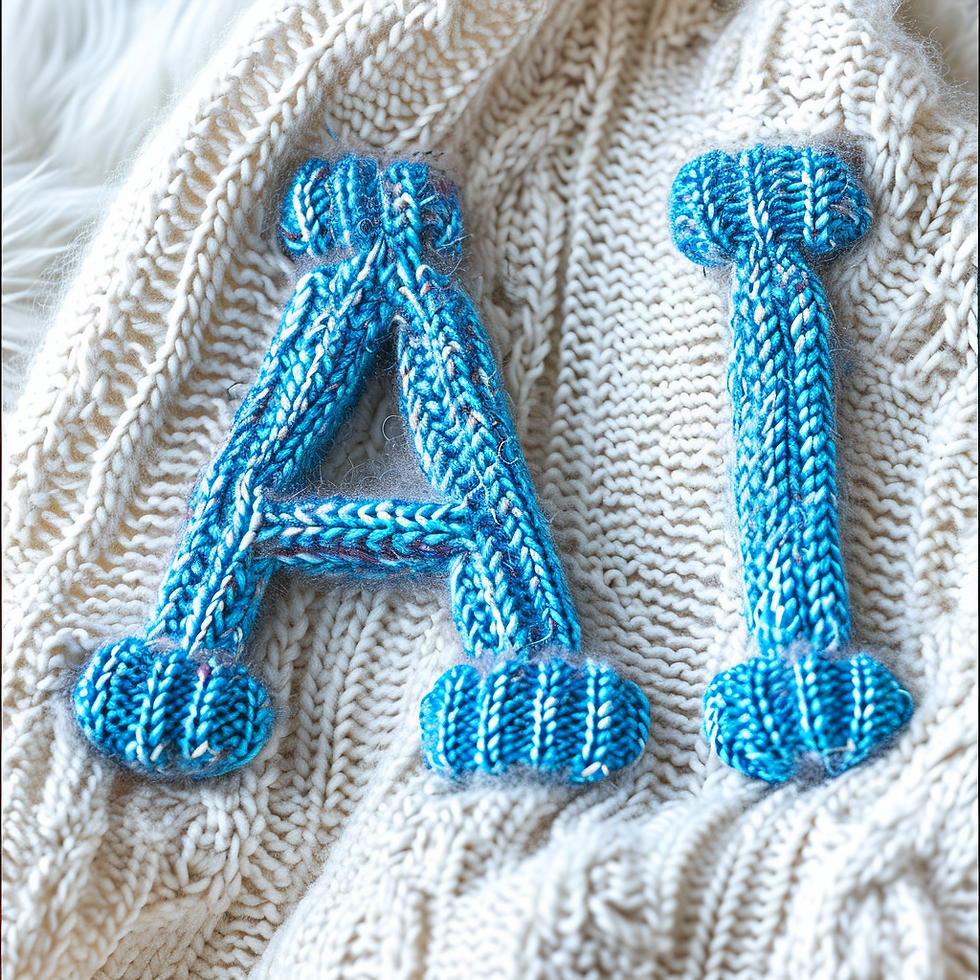
As businesses jump to embrace the transformative power of generative AI, one critical consideration often overlooked is the impact of tokens on cost. While the subscription models offered by major AI providers like OpenAI, Anthropic, and others may seem straightforward, the true cost of utilising these powerful language models may vary significantly based on factors such as the length and complexity of the inputs and outputs.
At the heart of this cost equation lies the concept of tokens – the fundamental units that make up the text data processed by language models. Tokens can represent words, punctuation marks, or even parts of words, depending on the tokenisation algorithm used by the model. The number of tokens in an input or output directly affects the computational resources required and, consequently, the cost incurred.
To better understand this relationship, let's consider a hypothetical scenario. Imagine a business user submitting a 500-word document to an AI language model for analysis or summarisation. While the subscription fee may appear reasonable, the actual cost could be significantly higher if the tokenised input exceeds the token limit included in the base subscription plan.
Moreover, the complexity of the task at hand can also impact token count. For instance, generating a concise summary from a lengthy document may require fewer tokens than producing an in-depth analysis or engaging in an extended dialogue. Understanding these nuances is crucial for businesses to accurately forecast and optimise their AI spending.
So, how can businesses effectively manage their AI costs while leveraging the power of these advanced language models?
Here are a few tips:
Tokenize and Analyse: Before submitting any input to an AI model, tokenize the text using the appropriate tokenization algorithm. This will provide an accurate token count, allowing you to estimate the potential cost and adjust your approach accordingly.
Optimize Input and Output Lengths: Whenever possible, aim to keep inputs and outputs concise and focused. Lengthy prompts or outputs can quickly escalate token counts and associated costs. Encourage users to be selective and prioritize the most essential information.
Leverage Caching and Batching: Many AI providers offer cost-saving options for caching and batching requests. By caching frequently used inputs or batching multiple requests together, businesses can reduce the overall token count and associated costs.
Implement Cost Monitoring and Alerts: Establish robust cost monitoring mechanisms to track AI usage and associated expenses. Set up alerts to notify stakeholders when costs approach predefined thresholds, enabling proactive cost management and optimisation.
Explore Alternative Pricing Models: As the AI market matures, alternative pricing models may emerge, such as pay-per-use or volume-based discounts. Continuously evaluate and compare these options to find the most cost-effective solution for your business requirements.
By understanding the role of tokens in AI cost structures and implementing strategies to optimise token usage, businesses can unlock the full potential of generative AI while maintaining control over their AI spending. As these powerful language models become increasingly integrated into various business processes, mastering token management will be a critical competitive advantage.
~ A ripe fruit will drop when it notices an honest person ~ Nigerian proverb
Comentarios